How Predictive Analysis Can Save Your Fleet Operations
October 12, 2023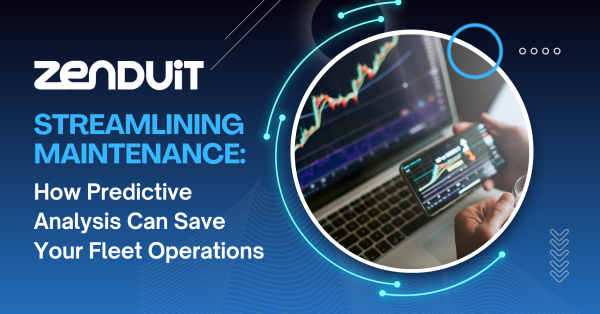
Traditional fleet maintenance strategies have often relied on a reactive approach, addressing problems as they arise. This method, while straightforward, comes with its own set of challenges. Unplanned downtime, unexpected repair costs, and the consequent disruption of services are just a few of the issues that fleet managers regularly face. Without predictive analysis, the volatility of these events can lead to inefficiencies and inflated expenses, significantly impacting the bottom line.
At its core, predictive analysis involves using data, statistical algorithms, and machine learning techniques to identify the likelihood of future outcomes based on historical data. In the context of fleet operations, this means analyzing vehicle data to predict maintenance needs before they become critical problems. This proactive approach stands in stark contrast to traditional methods, offering a way to anticipate and prevent vehicle failures.
The integration of predictive analysis into fleet maintenance can be transformative. By shifting from a reactive to a proactive maintenance model, fleet operations can achieve unparalleled efficiency and cost-effectiveness. The ability to predict and prevent issues not only minimizes downtime but also extends the lifespan of vehicles, optimizes resource allocation, and improves overall fleet performance.
In this article, we will delve into how predictive analysis is reshaping the landscape of fleet maintenance, turning challenges into opportunities for optimization and growth. Through a detailed exploration of its benefits, implementation strategies, and future potential, we will demonstrate that predictive analysis is not just a technological advancement but a strategic necessity for modern fleet operations.
Understanding Predictive Analysis
Predictive analysis is a forward-looking approach, utilizing data, statistical algorithms, and machine learning to forecast future events. It’s about sifting through historical and current data to find trends and patterns, then using those insights to anticipate what’s likely to happen next. This methodology is particularly powerful in fleet maintenance, where predicting the future condition of vehicles can lead to more efficient operations.
Definition and Explanation of Predictive Analysis
Predictive analysis is the process of using data to predict future events. It involves analyzing past and present data to identify trends, patterns, and potential risks. This approach is significantly different from traditional methods that rely on scheduled maintenance or fixing issues as they arise. Predictive analysis enables businesses to make informed decisions based on data-driven insights, leading to proactive rather than reactive strategies.
Key Components of Predictive Analysis in Fleet Maintenance
- Data Collection: This is the foundation of predictive analysis. Fleet vehicles are equipped with sensors and telematics devices that collect a vast array of data, including engine performance, fuel usage, tire pressure, and more.
- Data Processing and Analysis: The collected data is processed and analyzed to detect patterns and anomalies. This process often involves advanced algorithms and machine learning techniques to interpret large volumes of data efficiently.
- Predictive Modeling: Using the analyzed data, predictive models are developed to forecast future maintenance needs. These models can predict when a vehicle component might fail or when maintenance is necessary, based on the patterns observed in the data.
- Actionable Insights: The final step involves translating these predictions into actionable insights. This could mean scheduling maintenance activities before a predicted failure or adjusting fleet operations based on anticipated vehicle availability.
Examples of Predictive Analysis Tools and Technologies
- Telematics Systems: Telematics is crucial for data collection, offering real-time monitoring of vehicle performance and health. They provide the raw data necessary for any predictive analysis.
- Fleet Management Software: Such software often includes predictive maintenance modules. They can analyze telematics data to predict maintenance needs and schedule service proactively.
- Machine Learning Algorithms: These algorithms are used to process and analyze the collected data, learning from it to improve prediction accuracy over time.
- Data Visualization Tools: These tools help fleet managers understand the insights derived from the data, often presenting them in an easily digestible format like dashboards or reports.
- Integrated IoT Devices: Internet of Things (IoT) devices in vehicles provide additional data points, such as environmental conditions and operational patterns, enriching the data pool for more accurate predictions.
By harnessing these tools and technologies, predictive analysis enables fleet managers to anticipate issues before they occur, schedule maintenance efficiently, and avoid the downtime and costs associated with unexpected breakdowns.
Benefits of Predictive Analysis in Fleet Maintenance
Predictive analysis in fleet maintenance offers a myriad of advantages, fundamentally changing how fleet operations are managed. This approach enables a more proactive, efficient, and safe management of fleet vehicles, leading to significant cost savings and operational improvements.
Proactive Maintenance Approach: Anticipating Problems Before They Occur
Predictive analysis allows fleet managers to move from a reactive to a proactive maintenance approach. By predicting potential issues before they manifest, this approach enables timely interventions, preventing minor issues from escalating into major problems. This shift not only reduces the likelihood of vehicle breakdowns but also ensures that vehicles are always in optimal condition, ready to perform their duties without unexpected interruptions.
Cost Savings: Reducing Downtime and Repair Costs
One of the most immediate benefits of predictive analysis in fleet maintenance is the reduction in downtime and repair costs. Traditional reactive maintenance often leads to costly emergency repairs and significant downtime, as vehicles are unexpectedly taken out of service. Predictive maintenance, by contrast, allows for repairs to be scheduled during off-peak times, minimizing disruption and reducing the urgency—and often the cost—of repairs. Over time, this leads to substantial cost savings and more efficient use of resources.
Increased Lifespan of Fleet Vehicles: Extending Vehicle Usability Through Timely Interventions
Predictive maintenance extends the lifespan of fleet vehicles by ensuring that they are serviced at the optimal time. Regular maintenance based on predictive analysis prevents excessive wear and tear, addressing potential issues before they cause lasting damage. This not only extends the usable life of the vehicles but also enhances their resale value, providing a better return on investment.
Enhanced Safety: Preventing Accidents and Malfunctions
Safety is paramount in fleet operations, and predictive analysis significantly contributes to safer vehicles. By anticipating and addressing potential failures, predictive maintenance reduces the risk of accidents caused by vehicle malfunctions. This not only protects the drivers and the public but also helps in maintaining the company’s reputation and reducing liability.
Case Studies or Real-World Examples of Successful Predictive Maintenance Implementations
Here are some real-world case studies illustrating the successful implementation of predictive maintenance in fleet operations:
- Kanerika’s AI-Enabled Vehicle Breakdown Prediction Model: This model predicts when vehicles are due for maintenance by analyzing data from various sources such as sensor data, maintenance records, and driving patterns. The mobile application sends alerts to the driver, maintenance personnel, or fleet manager about vehicle maintenance needs, helping to avoid breakdowns, major repairs, and unexpected downtime. The results include reduced costs on repairs, improved safety by reducing the likelihood of accidents caused by mechanical failures, optimized fleet management, real-time monitoring, better warranty management, and improved customer service.
- Penske: The collaboration between Penske and their analysis solution led to the development of a predictive model called Proactive Diagnostics, using artificial intelligence (AI) and machine learning (ML). Penske can now flag vehicles with impending mechanical problems and repair them before they fail. This approach has reduced downtime, extended the life of assets, and supported higher levels of customer satisfaction. The Guided Repair solution is used by more than 9,700 Penske fleet maintenance technicians across more than 880 locations, addressing over 80,000 repair projects using this solution.
- Fox & James: Fox & James, a full-service truck leasing company, utilized an AI-powered platform to enhance their maintenance processes. The platform helped them improve quantitative reporting, enhance telematics alerts, eliminate redundant fault code analysis, and refine their processes. The key highlights include data-driven performance optimization, improved telematics alerts to anticipate and address vehicle issues before they become costly, and automation to enhance efficiency and reduce data discrepancies.
These case studies demonstrate the wide-ranging benefits of predictive maintenance in fleet operations, including cost savings, enhanced safety, optimized fleet management, and improved customer satisfaction.
Implementing Predictive Analysis in Fleet Operations
Transitioning to predictive analysis in fleet maintenance is a strategic move that requires careful planning and execution. Here are the key steps to effectively implement this transformative approach:
- Assessment of Current Operations: Begin by evaluating your current maintenance practices. Understand the limitations and identify areas where predictive analysis could bring improvements.
- Setting Goals and Objectives: Define clear, measurable goals for the transition. This might include reducing maintenance costs, decreasing vehicle downtime, or improving safety.
- Technology Selection: Choose the right predictive analysis tools and technologies that align with your fleet’s needs. Consider factors like the size of your fleet, types of vehicles, and specific maintenance challenges.
- Data Infrastructure Development: Develop a robust data collection and management system. This might involve upgrading existing telematics systems or installing new sensors and IoT devices in your fleet vehicles.
Integrating Data Collection and Analysis Tools into Existing Fleet Systems
- Seamless Integration: Ensure that the selected predictive analysis tools can be seamlessly integrated with your existing fleet management systems. This minimizes disruptions and facilitates a smoother transition.
- Data Quality Management: Implement processes to ensure the quality and accuracy of the collected data. Reliable data is crucial for effective predictive analysis.
Training and Development for Staff
- Training Programs: Conduct comprehensive training programs for your team, including fleet managers, maintenance technicians, and drivers. They should understand how to use the new tools and interpret the data.
- Continuous Learning: Encourage a culture of continuous learning and adaptation. As predictive analysis evolves, staying updated with the latest trends and technologies is vital.
Measuring and Evaluating the Effectiveness of Predictive Maintenance
- Performance Metrics: Establish metrics to measure the effectiveness of predictive maintenance. This could include metrics like reduction in downtime, maintenance cost savings, and improvements in vehicle longevity.
- Regular Reviews and Adjustments: Regularly review the performance against set metrics. Be prepared to make adjustments to strategies and tools based on these evaluations.
- Feedback Mechanism: Implement a feedback mechanism to gather insights from staff and drivers. This helps in identifying any practical challenges and areas for improvement.
By following these steps, fleet operations can smoothly transition to a predictive maintenance model, harnessing the power of data and technology to drive efficiency and reliability in their operations.
Challenges and Considerations
While predictive analysis offers significant benefits for fleet maintenance, implementing this approach comes with its own set of challenges and considerations.
Addressing Potential Barriers to Implementation
- Cost: Initial investment in predictive analysis tools and technologies can be substantial. It’s essential to consider the long-term ROI and plan for budget allocation.
- Resistance to Change: Shifting from a traditional maintenance approach to a predictive one may encounter resistance from staff. Effective communication and training can help in managing this transition.
- Technology Integration: Integrating new technologies with existing systems can be complex. It requires careful planning and expertise to ensure compatibility and seamless operation.
Balancing Predictive Analysis with Human Judgment
While predictive analysis provides valuable data-driven insights, it’s crucial to balance these with human expertise and judgment. Fleet managers and technicians should use the data as a tool to aid decision-making, not as a replacement for their professional judgment.
Ensuring Data Security and Privacy
With the increased use of data, ensuring its security and privacy becomes paramount. This involves protecting data from unauthorized access and ensuring compliance with data protection regulations.
Keeping Up with Technological Advancements
The field of predictive analysis is rapidly evolving. Fleet operations must stay abreast of new developments and continually assess and update their tools and strategies accordingly.
Customization to Fleet Needs
Predictive analysis solutions should be tailored to the specific needs of the fleet. This customization is key to maximizing the effectiveness of predictive maintenance strategies.
The Future of Fleet Maintenance
Looking ahead, fleet maintenance is poised to be revolutionized by continuous advancements in predictive analysis. This evolution is not just a trend but a paradigm shift in how fleet operations will be managed.
Emerging Trends and Innovations in Predictive Analysis for Fleet Maintenance
- Advanced AI and Machine Learning: These technologies will become more sophisticated, allowing for even more accurate predictions and automated maintenance scheduling.
- Integration with Autonomous Vehicles: As autonomous vehicles become more prevalent, predictive maintenance will play a crucial role in ensuring their reliability and safety.
- IoT and Connectivity: Enhanced IoT integration will lead to more detailed and real-time data collection, further improving predictive analysis capabilities.
- Sustainability Focus: Predictive maintenance will increasingly incorporate sustainability considerations, helping fleets reduce their environmental impact.
- Customized Predictive Models: Models will become more tailored to specific fleet types and operational needs, improving their effectiveness.
Predicting the Long-Term Impact on Fleet Operations and Industry Standards
- Standardization of Predictive Maintenance: Predictive analysis will likely become a standard practice in fleet maintenance, setting new industry benchmarks.
- Reduction in Operational Costs: Long-term, fleets that adopt predictive maintenance can expect significant reductions in operational costs and improvements in efficiency.
- Enhanced Fleet Safety and Reliability: Predictive maintenance will contribute to safer and more reliable fleet operations, reducing the risk of accidents and breakdowns.
- Data-Driven Decision Making: Fleet management will shift increasingly towards data-driven decision-making, optimizing operations across the board.
Final Thoughts
It’s clear that predictive analysis in fleet maintenance is not just a technological upgrade; it’s a strategic revolution. This approach offers key benefits: proactive maintenance, cost savings, increased vehicle lifespan, and enhanced safety. These advantages collectively transform fleet operations, driving efficiency and reliability.
Adopting a proactive maintenance strategy, underpinned by predictive analysis, is more than an operational choice; it’s a commitment to operational excellence. Embracing this technology empowers fleet managers to make informed, data-driven decisions, ultimately leading to more effective and sustainable fleet management. The future of fleet operations is undoubtedly intertwined with the advancements in predictive analysis, heralding a new era of smarter, safer, and more efficient fleet management.
Want to learn more? Contact your ZenduiT consultant today.